How to take advantage of network effects for heavy industry
Network effects and automatic selection are changing the AI game.
AI models for heavy industry are unique. They need the ability to function remotely, be resilient to all types of environmental conditions, and when required, work in real-time. In short, there’s a hurdle or three.
Another consideration is the fact that ‘heavy industry’ is a big catch-all. Think mining, construction, manufacturing, logistics, forestry and agriculture. Reliable AI is essential in these high-risk environments, yet the challenges of each are nuanced. Supporting workers at an open pit mine looks different from a warehouse. Not to mention, if that mine pit is in a remote location and has real-time AI requirements.
At Presien, we’re increasingly presented with unique AI vision challenges. We’ve learned that bigger datasets don't always equal better results when it comes to edge computing. Edge models have fewer parameters meaning the dataset must be more carefully selected. In these scenarios, data quality is just as important as quantity.
So how can heavy industry tackle capturing good data, plus enough of it?
A network to support success
When it comes to training robust AI models, variety is key. Snippets of data from multiple sites will build a much richer dataset than extensive data from one site. The more diverse the inputs, the more vivid the picture.
Let’s put this into practice with the example of AI vision. At Presien, we’ve built an AI vision system for heavy industry, called Blindsight. In our experience, a limited number of Blindsight devices distributed across a large number of sites will produce richer data than a single site with a higher concentration of sensors. This is because a wider selection of sites introduces more environmental variation. One device might be subject to a dusty setting, whereas another placed at a high point of a site may encounter different lighting.
This brings us to the Network Effect. A phenomenon whereby the more people use a service, the better it performs for everyone. The internet is a great example of the network effect, it grows in value the more people use it.
Keeping with this theory, the more sites that adopt our AI vision devices, the better and more representative our dataset becomes thanks to diverse environments, objects and camera angles. Dataset diversity is what drives down sources of error in our model, but how do we know this is working?
One way to measure this effect is to look at the number of errors our model makes on a heavy industry-specific dataset with accurate manual labels. There are two main types of error that this type of model can make: false positives and false negatives. False positives are instances where the model saw something that wasn’t really there. Reducing their occurrence is important to avoid unnecessary distractions but it must not come at the cost of increased false negatives. False negatives are instances when the system fails to detect something which is present.
Measuring both these types of error is valuable but only possible when we have accurate labels to begin with. Another way to see the impact of improvements to our model is to look directly at the number of alerts produced per hour.
The figure below shows that as the number of collective machine hours seen by our Blindsight system has increased, the number of alerts per hour, false positives and false negatives have all decreased. This is the network effect in action.
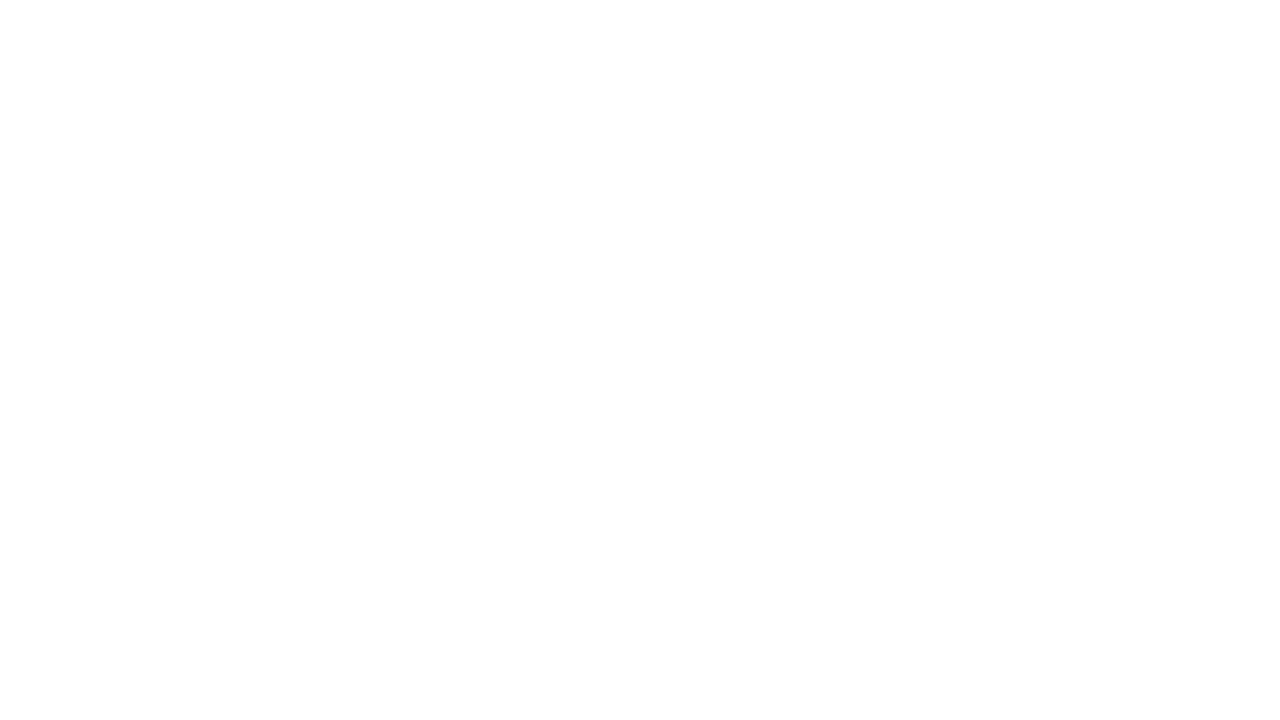
Over time this network can build a powerful resource: the data lake. A valuable archive of information where a high-quality dataset can be shaped and refined.
Data quality that scales
When it comes to building AI, mass data = mass labeling. For many teams, this is simply impractical. Also, not every piece of data is as useful as the next so automated techniques are needed to make sense of the data deluge.
Deciphering higher-quality examples from lower-quality inputs is crucial to building a scalable model. At Presien, we make use of active learning techniques to select high-value images to grow our data lake. These images can then be labeled and used to improve our existing models or to build new models for specific use cases.
We can also leverage our data lake to resolve specific issues with our models. For example, if there is a persistent type of false positive, few-shot learning can be used to search through the data lake for similar examples. These examples can be labeled and used to retrain the model to perform better on this type of object.
With tens of thousands of real-world images being processed by our active learning AI system every month, our model is continuously improving and adapting.
To recap, when trying to deploy AI in a heavy industry context there are numerous issues to overcome. In the case of AI vision, a shortcut to success is leveraging the network effect and employing automated techniques to secure the highest quality data.
Want an even quicker path to AI vision success? Presien’s existing model is embedded with 100,000 hours of development time and more importantly reliable detections.